MiaRec Big CX Update [Including Video]
BIG CX UPDATE | MiaRec
Tatiana Polyakova, COO of MiaRec, joins Kieran Devlin of CX Today for the Big CX Update to talk about:
🆕 The new products and features coming to MiaRec
🏆Transforming quality management in the contact center
🤖 Leveraging generative AI and Machine Learning in the contact center
Polyakova provides an insightful look at the next 12 months of MiaRec’s products and features roadmap, and the valuable benefits to customers and partners that they entail.
To view the the full interview, please watch the video below:
Read the full interview transcript below:
Kieran: Hello, and welcome to CX Today. My name is Kieran, and this is the latest in our Big CX Update series. In this session I am delighted to be joined by Tatiana Poliakova, COO at MiaRec. Tatiana, thanks for joining us.
Tatiana: Thank you for having me, Kieran.
Kieran: Great! Well let's get started. Can you give us some insights please into MiaRec’s product roadmap for the next year or so?
Tatiana: Absolutely! I'm very excited to share some major updates MiaRec’s team is currently working on. In the upcoming months our customers will see more product updates and features. All of those updates are centered around our commitment to helping contact centers to automate and scale quality management and compliance processes using AI.
The first major update I would like to talk about is what we are currently working on, applying a generative AI to our Auto QM, Automated Quality Management solution. But first, I would like to talk about what Automated Quality Management solution is and what are the use cases of Automated Quality Management in contact center environment. Let's start by talking about Manual Quality Management. Manual Quality Management in contact centers looks like supervisors listening to agents and filling out some scorecards which are basically questionnaires. They have to listen to the calls and answer some questions like, did the agent say, ‘Thank you for calling’, did the agent introduce himself and the company or say that the call is being recorded? This is a very tedious and time-consuming process. We estimate that on average a contact center manager spends 50 hours a month on manual evaluation. This is a lot of time, money, and labor costs. This is 600 hours per year and quite expensive. If we're talking about labor costs and let's say, just for example, the hourly rate is $30 per hour and we multiply it by 600 hours, it's going to be $18,000 just for one supervisor. If we are talking about a contact center with 10 supervisors, it's going to be $180,000 per year! What Auto QM does is eliminate those costs by automating the process. The software looks for keywords and phrases in transcripts and fills out the automated quality management scorecards automatically. This is a great approach. There are basically two approaches some vendors use. Some do very basic keyword searches, others, like MiaRec, support complex queries like find word ‘Call’ near word ‘Record’, in the beginning of the conversation and we can tell the system to look at the agent sides of the conversation only. This is a great approach and again it saves a lot of time and money, but there are some downsides. It's very time consuming. What we do at my MiaRec, we create very complex queries for the system to work, to create and customize those queries. We want to take it a step up and apply genitive AI to this process, to this technology, because genitive AI, and Chat GPT is a great example, is really great at the question answering tasks. You can ask AI to evaluate the agent for you and it's going to save you a lot of time and money, but also eliminates the need to create those complex queries. Our contact center customers can reap the benefits of auto scorecards as in our ROI use cases right out of the box. We're very excited about this feature. Again, we are committed to helping our customers to eliminate this manual process.
Now the next big update I would like to share with you today is an update to our other feature, our other functionality. It's automated data redaction functionality. At MiaRec, we are currently working on AI based automatic data reduction functionality which is based on Named Entity Recognition (NER) technology. Let me explain what named entity recognition is in a moment. First, I would like to talk about some use cases for our contact center customers and recognize the importance of data reduction in contact center environments. To be compliant with legal requirements we must remove certain information for privacy concerns such as credit card or Social Security numbers. They cannot be collected, recorded, or stored in call recordings and transcripts. At MiaRec we have AI-Powered Automated Data Reduction functionality that automatically removes this private information from your call recordings, from contact center recordings, and transcripts. This can be done in three possible ways. The first, a very simple approach is to redact all numbers, but you cannot do much with those recordings after that or with those transcripts. You cannot analyze this data. If you remove, let's say, a credit card number and the customer accounts number the use of this data will be questionable. What we do with MiaRec is create those complex queries to look for key phrases like, ‘What is your credit card number?’. It is important to mention that the agent doesn’t necessarily say the phrase as it is. It can be, ‘Whenever you're ready, please tell me your credit card number’, and different variations of that. In order to provide the most accurate solution for our customers, MiaRec will create very complex queries looking for all variations. What are the key phrases or what are the keywords agents will mention in the in the conversation that will be before the credit card number? Again, this is a great approach, but we would like to take it to the next level. The approach we're going to use and what our team is currently working on is machine learning. We're going train a special model called Named Entity Recognition (NER) on many hundreds or even thousands course examples. During the recent development of Natural Language Processing (NLP) such approach provided really good results. The model understands the context on the conversation so it can predict with high accuracy if those digits belong to a credit card number and must be redacted or maybe those digits are phone numbers or account numbers that you would like to keep in the recordings. There are two big advantages to this matter and this technology. It's very robust and it understands context, just like in our example with genitive AI. The second great benefit is our customer contact center customers will be able to use Auto Data Reduction right out of the box without spending so much time on customer customization and onboarding while we’re creating those complex queries for them.
Kieran: Brilliant, yeah. That's really interesting. Thanks, Tatiana. I think it's remarkable all the different use cases that AI, especially generative AI is providing and you know in analytics and contact centers. I think that's very comprehensive. Thank you so much. I think that's maybe a great place to end it. It has been a really good session, so thank you Tatiana for being here today.
Tatiana: Definitely. Thank you, Kieran. It was my pleasure.
Kieran: If you enjoyed this video, please give us a like and share on social media and we'll see you next time. I’m Kieran Devlin of CX today. Thanks for watching.
Share this
You May Also Like
These Related Stories
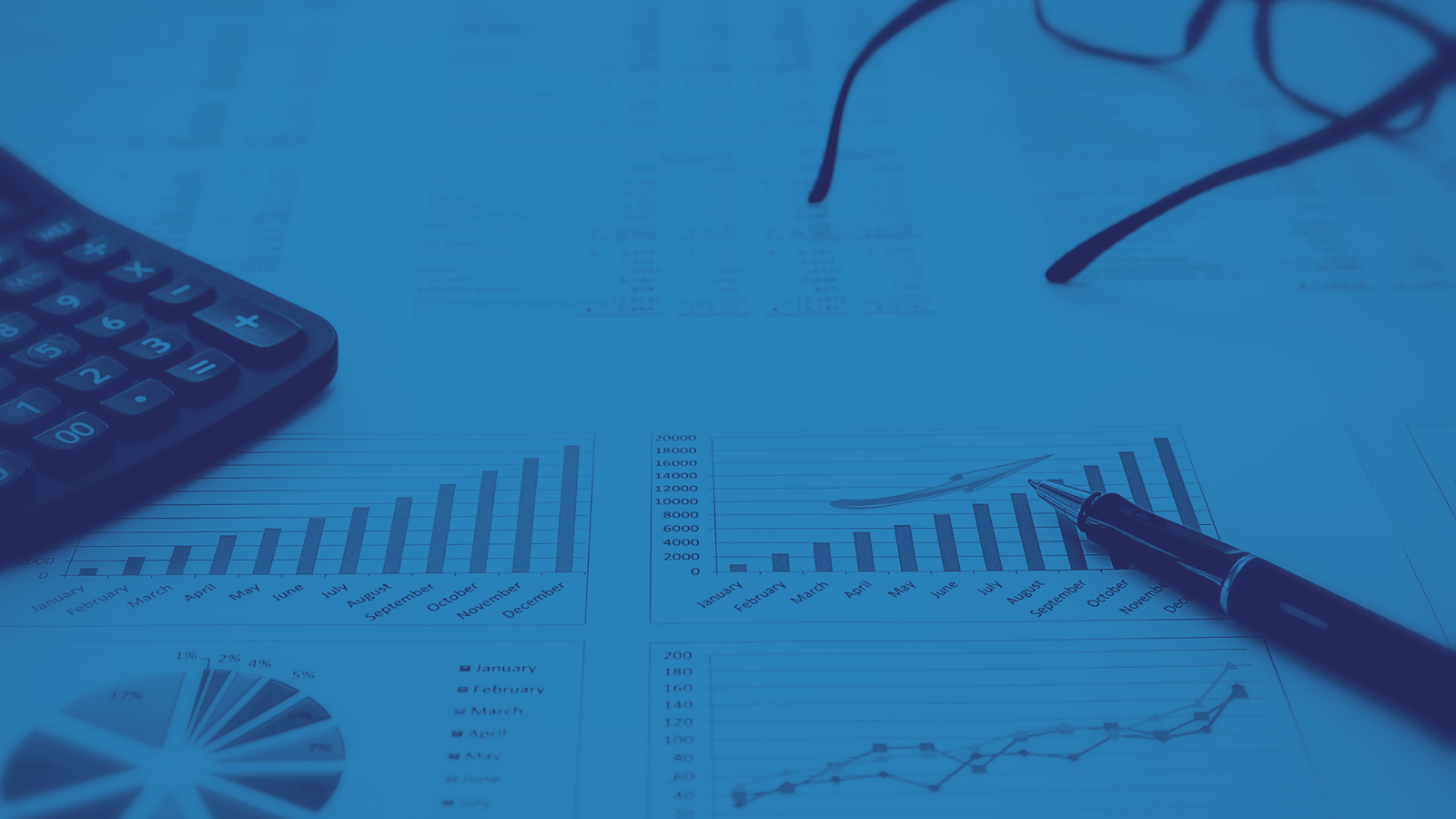
Tips On How To Maximize Your Call Center ROI
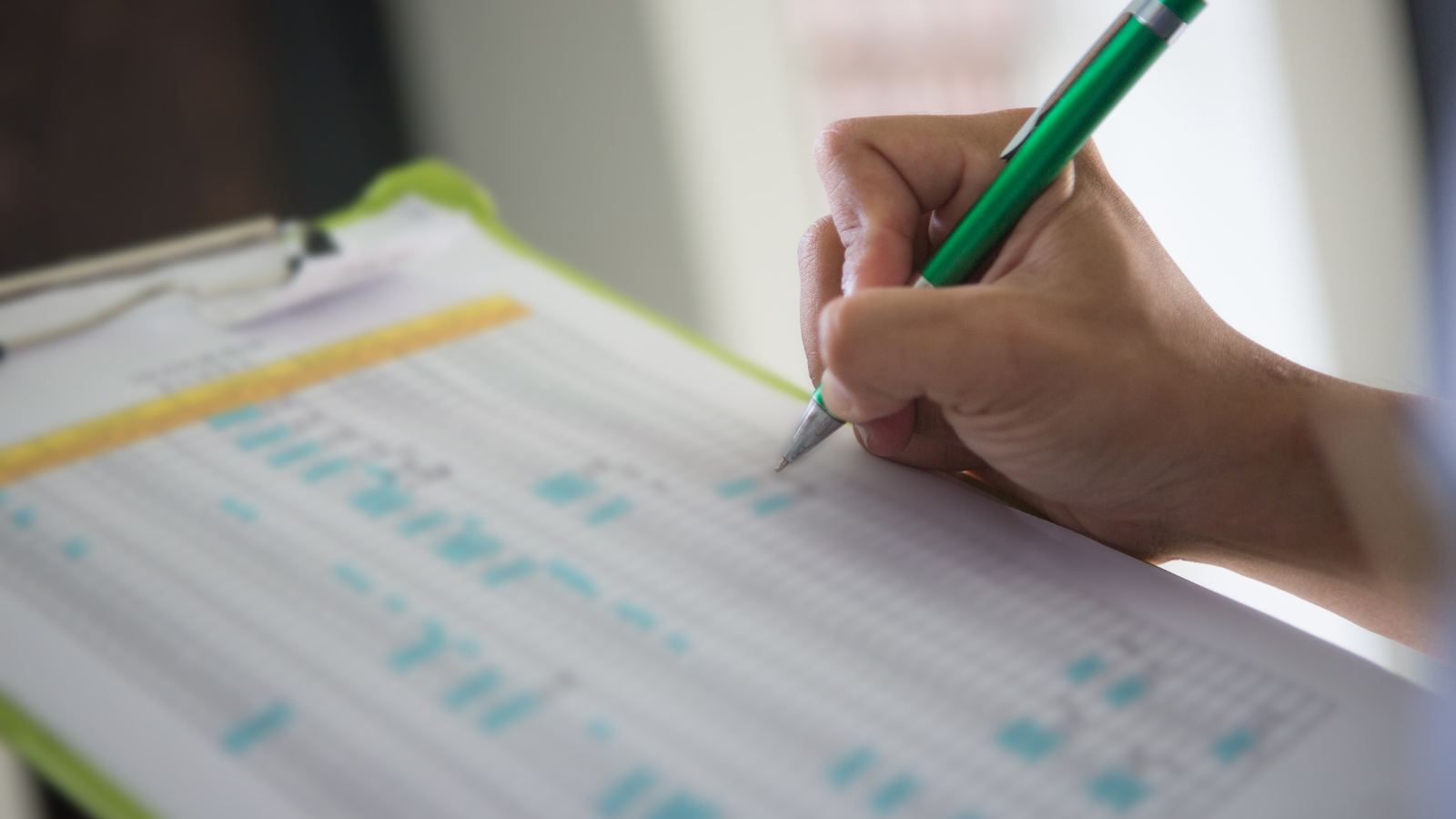
How To Use AI To Drastically Improve Contact Center Script Adherence
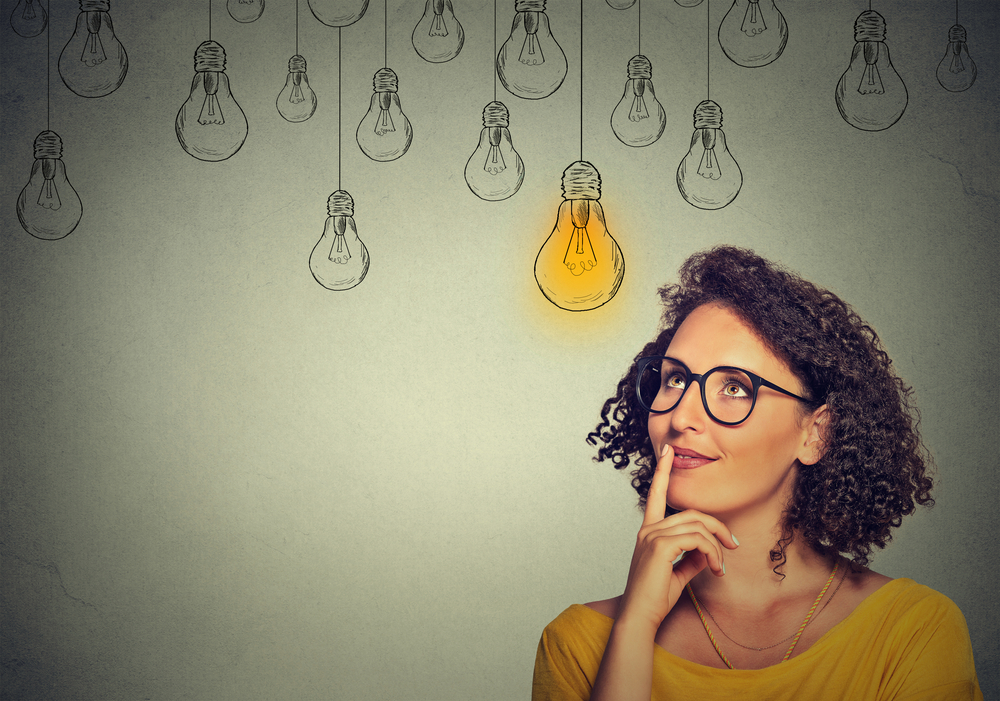